The art and science of prevention — Real-time system identification for smart maintenance
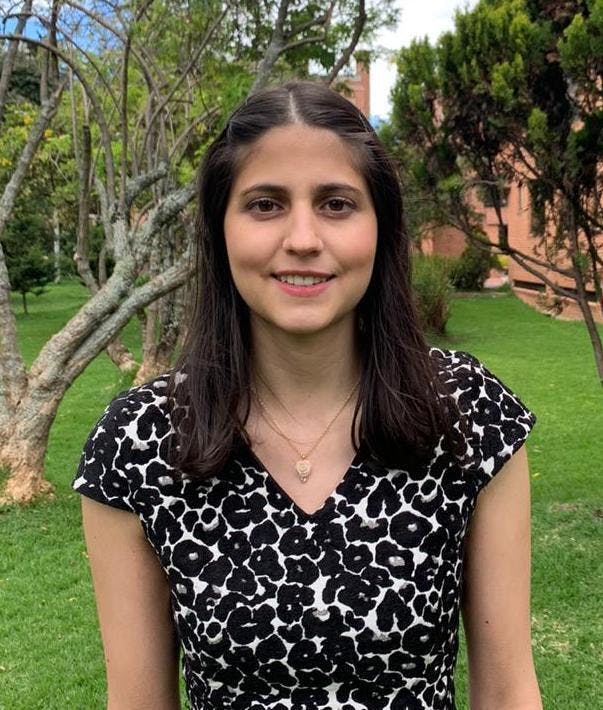
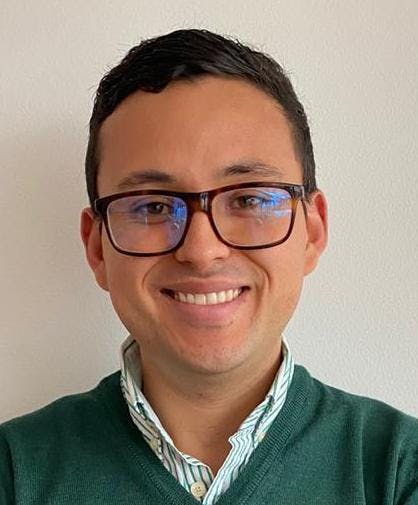
Paula Pico
Juan Pablo Valdes
Overview
This blog post highlights the advantages of bringing together real-time data, system identification methods, and data-driven models to develop smart maintenance plans for physical assets and manufacturing processes. The blog aims to disentangle the seemingly intricate and convoluted steps required to design a preventative maintenance framework, touching on its tangible benefits through industrial case studies. The blog is aimed at both business leaders and practitioners looking to make the case for advancing predictive maintenance and adoption of data streaming within their organisations.
System identification via stream processing
Continuously generated data or ‘data streaming’ has become ubiquitous in our day-to-day lives. Highly interconnected working environments such as financial trading floors or e-commerce platforms and entertainment services like Netflix or Spotify heavily rely on data streaming. Companies are relying on data being streamed back to them to predict market and consumer behaviour.
Consequently, streaming analytics is receiving attention due to wide availability of cheap sensors, larger computing power including edge devices and cheap cloud storage. Consequently, there is renewed focus on newer software infrastructure and novel algorithms to unlock the enormous value behind the patterns and trends held within the datasets generated by streaming devices. But what is streaming analytics?
Streaming analytics: At the very core, streaming analytics refers to a type of data analysis where real-time acquired data is handled and processed continuously (in real time or near real time) with continuous update of the outputs for further downstream processing or visualisation. This analysis type goes a step further than traditional batch processing of historical data offline.
Early on, social networking services exploited the power of streaming analytics by gathering and understanding real-time trends. Multiple metrics, such as website clicks, user traffic influx and geo-location, dwell time per product/section, allow companies to react timely to external factors such as shifts in consumer interest. The information that you clicked on all those cat pictures is being streamed to an AI algorithm which is helping your social media provider suggest more funny cat videos back to you.
Other interesting non-social media uses of streaming analytics are:
- Transportation, manufacturing and industrial processing: preventative maintenance, fault detection and prognosis using data streams from equipment sensors.
- Healthcare: real-time monitoring of patient’s condition, vital signs, and relevant metrics, contributing to a real-time clinical risk assessment.
- Finance: tracking fluctuations in stock market prices and other external factors to rebalance portfolios from real-time value-at-risk analyses.
In the context of heavy industry, reduced downtime, lower costs and effective maintenance planning are all being made possible by the ability to detect anomalies in a timely fashion. Engineers are increasingly seeking to augment their predictive capabilities to promptly identify patterns which do not conform to expected behaviour. It is not a surprise that the concept of detecting anomalies has become quite popular in the aerospace and manufacturing sectors, where the ability to act proactively instead of reactively towards a faulty system or an inefficient maintenance schedule has proven to be vastly efficient and is saving a lot of time and money.
System Identification and its role in smart maintenance
Bridging the gap between observed data and descriptive mathematical models to make predictions is a foundational area in science and engineering. In the realm of control systems, this operation is referred to by the umbrella term System Identification (SystID), which, in its most fundamental form, is the process of constructing and building models of dynamical systems from observed input-output signals. In other words, SystID aims to identify and abstract the essential underlying dynamics and features of a system that bring about its outputs; as one might expect, SystID finds common ground with—and is indeed largely based on—statistical theory, data-driven decision-making, parameter estimation, black-, gray- and white-box modelling, and on(off)-line optimisation methods.
Online SystID frameworks have greatly improved prognostic maintenance and early failure detection. Prognostic maintenance is defined by DNV—an assurance and risk management company based in Norway—as “seeing into the future” 1 by preemptively and proactively probing the present condition of a system, identifying potential failure points in real-time, and planning and scheduling maintenance operations before the threat materialises into a critical—and oftentimes exceedingly costly—failure in a real-life scenario. In colloquial terms, this translates into a “prevention is better than cure” or “better safe than sorry” philosophy. Failure detection is related to the inspection of the systems’ dynamic behaviour and the identification of the signs and patterns characteristic of divergence from normal operation, as well as the timely alerting of these operational abnormalities.
A ‘smart’ failure detection and maintenance system goes beyond traditional preventative maintenance methods and uses an integrated approach whereby the insights gathered from multiple sources (e.g., on-site and historical measurements, physics-based simulations, and data-driven models) are connected, continuously updated, and streamed to develop maintenance plans to prevent downtime, increase process and assets reliability, facilitate risk assessment, and accelerate maintenance operations. Industrial manufacturers are estimated to sustain annual monetary losses of about 260,000. McKinsey & Company has documented the >50% decrease in downtime2 of rotary equipment in an energy-related operation attained by developing a complex predictive maintenance algorithm with physical-dependent parameters, saving millions of dollars per maintenance instance3. The aviation industry in 2018 reported losses of $20 billion due to unplanned maintenance operations, which amounted to a whopping 27% of all maintenance costs that year. Using data-powered analytics, time-series analyses, and physics modelling for smart maintenance scheduling, Emirates reported a 56% reduction in unexpected engine removals4, saving costs and increasing flight safety. Despite these successes, industries face enormous technical and technological challenges when adopting optimisation-based preventative maintenance and failure detection practices. This is due to the complex interplay between subsets of industrial-scale systems that requires the acquisition of state-of-the-art sensors, complex connectivity systems, optimisation algorithms, and even data security protocols. In addition, the substantial upfront costs and efforts associated with setting up and building an integrated predictive maintenance and failure detection framework, including personnel training, are a major deterrent to some organisations.
Annual industry impact
- $50 billion losses due to unplanned downtime
- $20 billion losses due to unplanned maintenance
Even with these challenges, many companies across a spectrum of fields have leveraged heavy investments in infrastructure to deploy prognostic and preventative maintenance practices, but have not yet been able to extract their full potential. In practice, a small percentage of the available infrastructure for these systems has been fully activated or properly tuned for each specific set of inputs and constraints. Moreover, the companies who do manage to fine tune, lack sufficient flexibility and robustness to promptly identify operational ‘red flags’ (i.e., forecast process failure) and re-adjust the system’s configuration efficiently. This due to the absence of connectivity between data acquisition and real-time processing (i.e. streaming analytics).
The lack of connectivity between data acquisition and streaming analytics is prohibiting companies to promptly identify operational ‘red flags’ (forecast process failure). Even so, many investments in infrastructure to deploy prognostic and preventative maintenance practices is well under way. But how can companies extract their full potential of such investments?
Real-time SystID in Quaisr
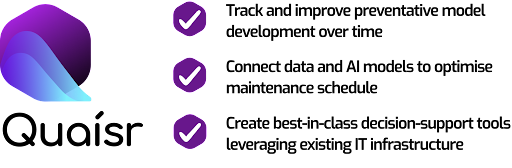
As the saying goes, “a tool is only as good as the hands that wield it”. In our current day and age however, it is not just a matter of how good the “hands” are anymore, but how freely available they are to make the most out of the tools at their disposal. Instead of letting engineers plummet into an endless-yet-necessary ocean of laborious tasks, they can take the wheel behind the crucial decision-making processes by handing over the background tasks and computations to powerful AI technology, which can improve, speed up and automate repetitive data processing, all while reducing human bias at the same time. Such is the case with SystID for preventative maintenance.
Today, data-driven algorithms can be readily used to develop robust predictions of possible failure scenarios and lay-out an efficient plan for downtimes and inspections that minimises operational impact.
Today, SystID computations can be performed on both historical and real-time data, ensuring that the system is highly responsive to external perturbations.
Today, the Quaisr platform speeds up the adoption of SystID and empowers experts to quickly advance efforts in areas efficiency and reliability.
The good news?
- No need for a steep and costly learning curve with extra steps or in-between setups to fully integrate real-time data acquisition with advanced analytics.
- The Quaisr platform offers a seamlessly connected and collaborative workflow environment where in-situ data can be streamed directly to both open-source libraries (SIPPY) and commercial SystID options (MATLAB). Using physics-driven process models is also within the scope of the Quaisr platform.
The best part?
- Leverage your existing IT infrastructure without upskilling in deployment. Quaisr navigates IT complexity so you can focus on being engineers and scientists! It is as simple as creating and connecting blocks in a drag-and-drop environment (with the additional flexibility of customising your own algorithm if you are an expert user).
- Quaisr is completely model agnostic, as it is inherently built to work around any type of library (both commercial and in-house custom code), meaning SystID algorithms can be smoothly replaced/updated without any major modifications to the workflow. Engineers can develop with confidence over time.
- Connectivity carries many other advantages. SystID processes can be coupled with machine learning and data-driven approaches to enhance model calibration and substantially speed-up computations. Quaisr is built to create, connect and consolidate blocks that will help you accelerate the digitalisation drive behind SystID workflows.
In our next blog article we explore what Quaisr can achieve with an example using the open-source SIPPY library for SystID.