Your digital twins must answer three fundamental questions
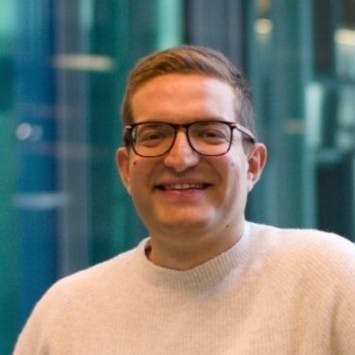
Assen Batchvarov
"Keep 'em Running," were the words of astronaut Gene Cernan when he entered the Apollo simulator room following the ominous message from the Apollo 13 crew – "Okay, Houston, we've had a problem here." Nearly 330 000 km away from Earth, the journey to the moon of James Lovell, John Swigert and Fred Haise was suddenly interrupted by the explosion and rupture of Oxygen Tank 2 in the spacecraft Service Module. With precious oxygen supplies leaking into space, there was little time to make critical decisions that would safely bring the three astronauts back home. Every decision had to be made with the certainty that it would not cause further damage, leaving the three astronauts stranded in space. The simulators that Cernan was referring to had been used for 30 000 hours to train astronauts and mission controllers; they were now a key technology that could safely return the crew back to Earth. By adapting the simulators using live telemetry data, Mission Control managed to explore navigation strategies well outside the spacecraft's original design envelope. The use of live data and simulations for the Apollo 13 crew's safe return to Earth is widely recognised as the first real-world use of digital twins.
The use of live data and simulations for the Apollo 13 crew's safe return to Earth is widely recognised as the first real-world use of digital twins.
It was years later that NASA formalised the definition of a digital twin in the 2010 Technology Area 12 Road Map (pdf). Nowadays, advancement in computing and sensor technology means that digital twins are no longer restricted to multi-billion-dollar space programmes. Digital twins are a transformative technology that is drawing together modern-day concepts such as Industry 4.0 and IoT with physical disciplines that involve modelling and simulation. Industries such as renewables, manufacturing, the built environment and many others are adopting digital-twin technology to help manage project lifecycles at all scales – from individual equipment to large-scale production lines and major infrastructure projects. In order to make a material difference to the safety, reliability, and profitability of their physical systems, digital-twin adopters are trying to answer three fundamental questions.
Advancement in computing and sensor technology means that digital twins are no longer restricted to multi-billion-dollar space programmes.
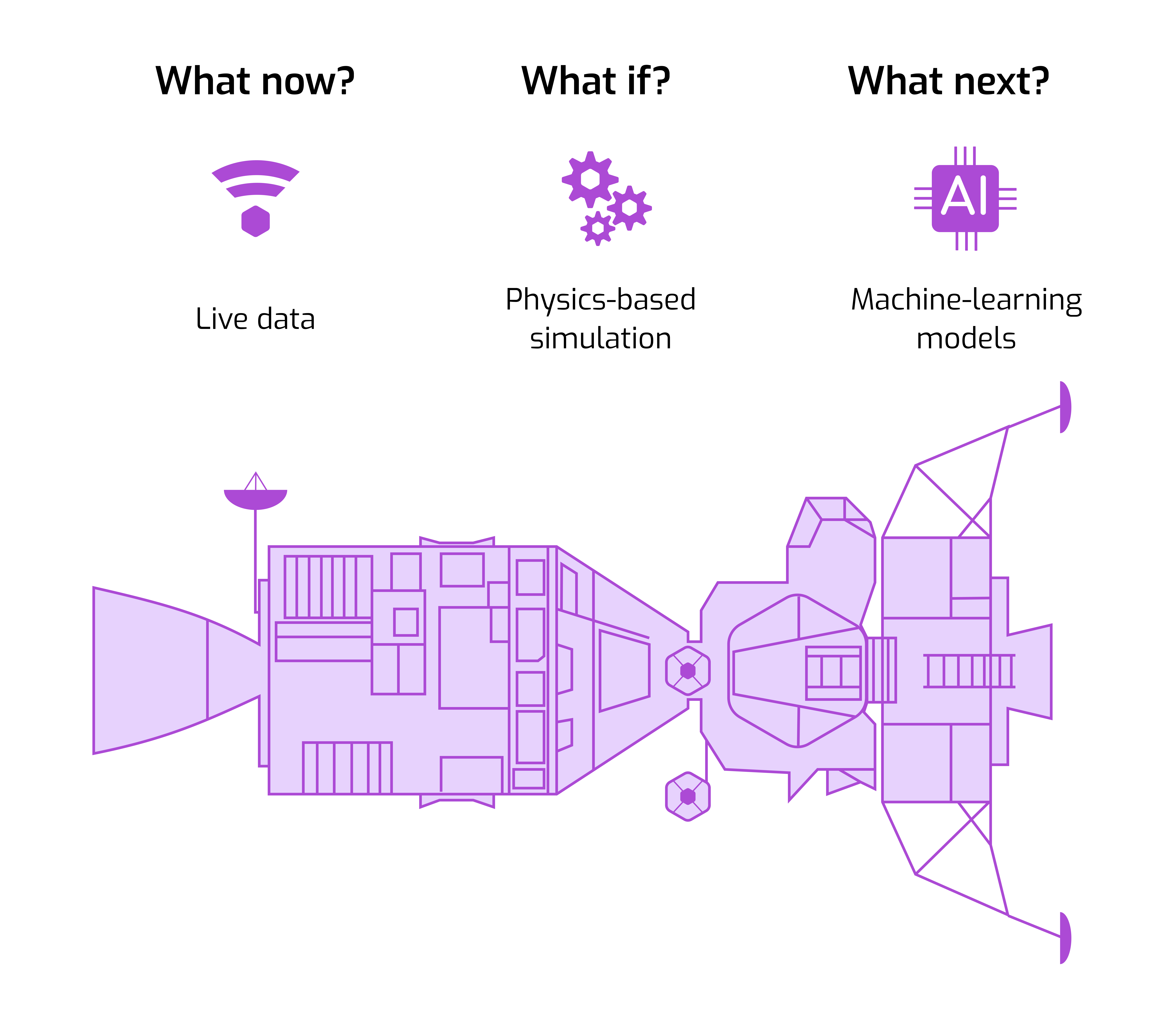
What now?
Knowledge of what is happening with your physical assets in real time can save lives. Live telemetry data alerted Mission Control to the failure of two fuel cells. Today, IoT streaming technology is widely available and allows companies to monitor systems in real time. Businesses can be alerted to critical problems remotely, paving the way for more efficient operations management, and assets with automation at the core of solution intervention. But as is the case with many complex systems, the data alone were not enough to return the Apollo 13 mission to Earth. Instead, the use of a digital twin, which supercharged live data streams with simulation capability, saved the lives of the three-member crew. The approach is being embraced rapidly by businesses: 85% of companies that have adopted IoT technology are implementing, or are about to implement, digital twins. Broad adoption shows that companies are realising the immense potential that the combination of live data with digital twins can have in their business. However, significant challenges still remain, with most companies yet to unleash the full potential of digital twins via, for example, incorporation of existing large-scale physical models and continuous calibration. As was the case for the Apollo 13 mission, answering "What now?" to diagnose the problem was only the first stage of the digital-twin solution framework.
What if?
The Apollo simulators were Mission Control's critical tool for stress-testing scenarios that would allow the crew's safe return. Answering "What if?" questions was critical for Apollo 13 operations, and instrumental in redesigning the spacecraft for the subsequent Apollo 14 moon landing. Simulations play a critical role during the design phase of present-day industrial projects. Construction, energy and infrastructure projects, manufacturing, healthcare, and many other industrial sectors trust complex physical models to provide critical information needed to build safe and reliable assets and systems. Nevertheless, with the adoption of digital twins, the role of physics-based frameworks is shifting from design-phase development and analysis to operations-phase monitoring, control, and decision support. Gartner claims that 48% of digital-twin adopters are sourcing data from computer-aided engineering (CAE) systems. Just as Mission Control were exploring unforeseen navigation strategies, adopters are using digital twins to extrapolate predictions beyond normal operational envelopes. Expedition into space uncharted by existing data is only possible with the coupling of mechanistic models and a digital-twin ecosystem.
The adoption of digital twins, the role of physics-based frameworks is shifting from design-phase development and analysis to operations-phase monitoring, control, and decision support.
The value of simulations extends beyond extrapolation: simulations are set to play a significant role in the data-centric engineering paradigm by offering a trustworthy foundation for optimisation, surrogate model development, data assimilation, data-driven model calibration, design of experiments, uncertainty quantification, and inverse problems.
What next?
To bring the crew back home, Mission Control had a critical task: determining what to do next. By exploiting a complex assembly of live simulators, they tested and rejected countless procedures until the final plan was clear. To keep the astronauts alive, whatever power was left would be spared; while the Lunar Module would be repurposed as a "lifeboat", and nursed back to Earth by Mission Control. With the recent democratisation of artificial intelligence (AI), business decision makers can now leverage their data and make predictions about the future. Purely statistical machine-learning models, or data-only approaches, have become the de facto standard in diagnosing rare diseases and planning advertising campaigns. In the physical disciplines, digital-twin adopters now see the consolidation of live data with machine-learning models as one way to answer "What next?" questions. However, there is a degree of scepticism amongst engineers: is machine learning alone sufficient to address complex industrial problems? Typically, domain specialists use mechanistic simulations to determine future outcomes in complex industrial settings.
Certainly one of the largest pitfalls of data-only approaches is the unreliability of models, particularly when exploring inputs beyond the training domain. Building machine-learning models using only observational data can also prove high risk, as in most cases operations data is noisy and unreliable – due to, for example, uncalibrated or faulty equipment. The only viable alternative is to move to data-centric engineering, where data-only approaches are complemented with simulations to guide the collection, generation, curation, and interpretation of data.
Quaisr allows data-centric engineers to incorporate simulation approaches into wider digital-twin ecosystems. We provide a framework for the "What if?" and we integrate it with the "What now?" and "What next?" to unlock truly predictive digital-twin solutions.